Manual fact-checking does not scale well to serve the needs of the internet. This issue is further compounded in non-English contexts. In this paper, we discuss claim matching as a possible solution to scale fact-checking. We define claim matching as the task of identifying pairs of textual messages containing claims that can be served with one fact-check. We construct a novel dataset of WhatsApp tipline and public group messages alongside fact-checked claims that are first annotated for containing “claim-like statements” and then matched with potentially similar items and annotated for claim matching. Our dataset contains content in high-resource (English, Hindi) and lower-resource (Bengali, Malayalam, Tamil) languages. We train our own embedding model using knowledge distillation and a high-quality “teacher” model in order to address the imbalance in embedding quality between the low- and high-resource languages in our dataset. We provide evaluations on the performance of our solution and compare with baselines and existing state-of-the-art multilingual embedding models, namely LASER and LaBSE. We demonstrate that our performance exceeds LASER and LaBSE in all settings. We release our annotated datasets, codebooks, and trained embedding model to allow for further research.
How to cite: Ashkan Kazemi, Kiran Garimella, Devin Gaffney, and Scott Hale. 2021. Claim Matching Beyond English to Scale Global Fact-Checking. In Proceedings of the 59th Annual Meeting of the Association for Computational Linguistics and the 11th International Joint Conference on Natural Language Processing (Volume 1: Long Papers), pages 4504–4517, Online. Association for Computational Linguistics. DOI: 10.18653/v1/2021.acl-long.347
We collaborated with 53 partner organizations worldwide to design and carry out our 2024 elections projects. We extend special gratitude to our lead partners in Brazil, Mexico and Pakistan, whose work we highlight in this essay.
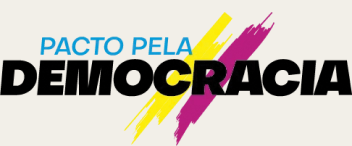
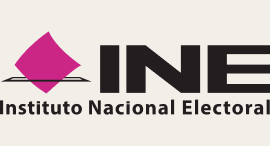
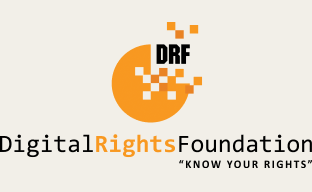
The 2024 elections projects featured in here would not have been possible without the generous support of these funders.

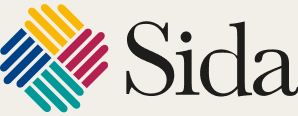
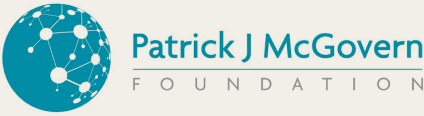
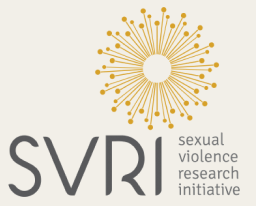
Footnotes
References
Authors
Words by
<p><a href="https://www.ashkankazemi.ir" title="Ashkan's personal website">Ashkan</a> is a natural language processing (NLP) intern at Meedan, contributing to research efforts in building fact-checking technology. He is also a PhD candidate at University of Michigan’s department of Computer Science and Engineering.</p>
Dr Scott A. Hale leads Meedan’s research in human-in-the-loop machine learning and natural language processing to create equitable access to information. He is a professor and researcher at the University of Oxford on the topic of hate speech, misinformation, and broadening access to data and methods.